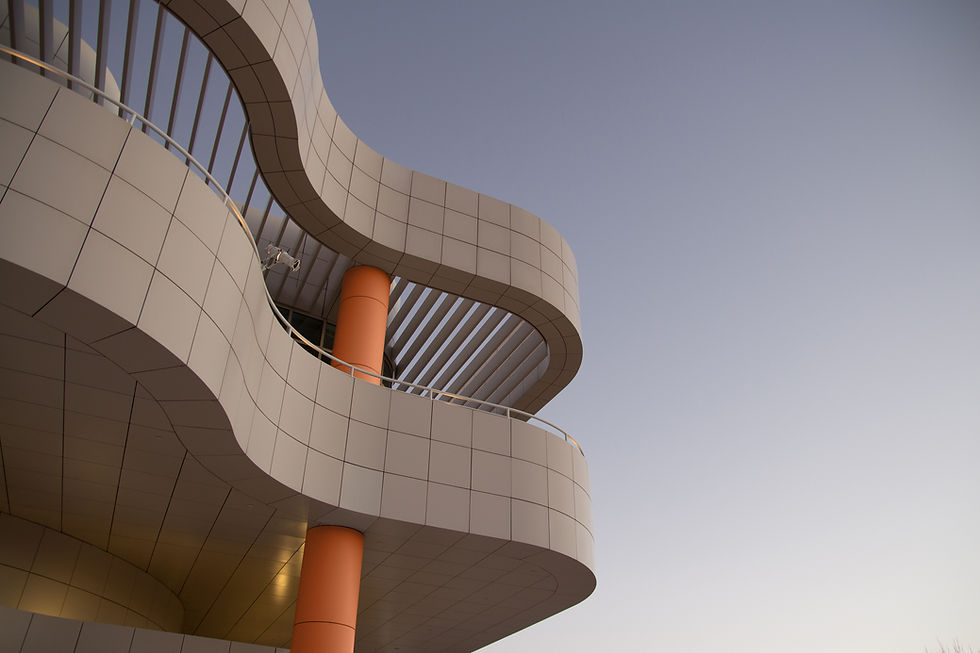
Pitfalls of Staying Too Long
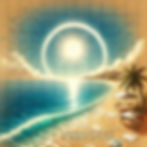
Ah, the problem space — what a wonderful place to be. It’s like planning a vacation that you’ll never actually go on, but the planning itself is so enjoyable that you almost don’t want to leave. You’ve got your metaphorical travel guide open (thanks ChatGPT), a luxurious itinerary mapped out, and a sense of adventure tingling at your fingertips. There are so many exciting possibilities: Should we go to that charming little town no one’s heard of (a niche market), or explore the bustling city of unsolved customer pain points? Or maybe we’ll just stay here a while longer in this luxurious, indulgent land of ambiguity, sipping on lattes and wondering what might be.
Before you know it, you’ve planned this epic, far-flung holiday, complete with daily activities, contingency plans for rain, and even a list of restaurants to visit that cater to every diet under the sun. But here’s the kicker: the trip never actually happens. You’re so caught up in the planning that the very idea of packing your bags and stepping outside feels… well, impractical. There’s always one more thing to think about, another route to consider, another delightful thought experiment to wander through.
And that’s exactly how staying too long in the problem space feels in a start-up. It’s warm, comfortable, and full of potential, but sooner or later, you’ve got to face the reality that at some point you actually have to pack up and go — build something, test it, and risk that the real world might not be as cosy as your imagined one.
So let’s leave behind the sun-drenched shores of the problem definition, where every wave of inspiration brings a new potential issue to explore. Let’s take a deep breath and dive into the customer validation ocean, where the reality check might be a bit colder but offers a clearer path. After that, we’ll pass through the treacherous yet essential lands of prioritised solutions, where everything can’t possibly get done, but what must get done rises to the surface. Then, there’s the hike through the hypotheses to test jungle, where the trail may be hard to follow, but it’s the only way to know if you’re headed in the right direction.
Finally, we arrive at the MVP — that minimalist destination that may not have all the creature comforts of our luxury problem-space resort but promises something infinitely more valuable: feedback, real-world data, and the next step forward.
Pack your bags. The problem space has been fun, but it’s time to head out.

Now that we’ve survived the choppy waters of the Customer Validation Ocean, it’s time to talk about when we first dipped our toes into the firmographic and tiering seas. These two mystical terms, once obscure and cryptic, now seem like keys to unlocking the mysteries of our customer base.
Imagine: we’ve taken the responses from our carefully crafted customer validation questionnaire (in our case about workflow automation) and, armed with firmographic wisdom, we start sorting through the data. Suddenly, the fog clears. No longer are we just looking at a random collection of people who happen to struggle with workflow inefficiencies. Now we see them as distinct species of businesses, each with their own characteristics, challenges, and - dare I say it - value.
Firmographic segmentation, if you’re still unfamiliar, is essentially the art of categorising companies based on traits like industry, company size, and location. It’s the kind of insight that makes you say, “Ah! So that’s why a scrappy, 10-person fintech start-up has very different needs from a global healthcare giant.” Who knew that understanding the size and sector of your target customer could actually help you shape your product? It’s like discovering that there are different types of shoes for different sports. Revelatory.
But wait, there’s more! Enter tiering, the secret sauce to customer prioritisation. Once we’ve got our firmographic categories in hand, we can start thinking about who matters most. Tiering lets us sort our customers by their value to us—whether that’s in terms of revenue potential, strategic importance, or even just how much they make us feel warm and fuzzy inside. Tier 1 is where the big fish swim, Tier 2 might be for those middle-market players, and Tier 3? Well, let’s just say they might still be using dial-up and fax machines, but hey, they’ve got potential.
In the context of our customer validation results, we’re looking at how different industries (financial services vs. education) responded to our questions about their workflow challenges. What’s that? You’re a multinational logistics firm, overwhelmed by manual data entry? Welcome to Tier 1 — we’ll be focusing on you! Oh, a local bakery struggling to automate their inventory? Love the ambition, but you’ll be in Tier 3 for now.
These insights tell us not just what our customers need, but how to prioritise who we help first, and - crucially - how we’ll make money along the way. Firmographics and tiering are like magic compasses, guiding us through the murky customer data and helping us spot the shiny opportunities that actually move the needle.
So now, thanks to these newfound tools, we’re not just surviving the customer validation ocean — we’re charting a course toward real, revenue-generating land. We can almost hear the sound of the MVP taking shape in the distance.

As we venture into the Hypotheses to Test Jungle, we’re now getting closer to something tangible — prioritised solutions that will guide us toward our MVP build. To find our way through, we need a clear structure for testing the assumptions we’ve gathered from our customer validation process. The key is to apply a simple, effective hypothesis framework that looks like this:
“We believe [this outcome] will happen if we do [this action] because [this rationale].”
This framework helps us identify what we’re trying to achieve, what action we’ll take to test it, and the reasoning behind that test. Let’s walk through a few prioritised solutions using this approach, considering tools like smoke tests and other practical methods to validate these hypotheses. Remember that we will supply workflow automation solutions and this is the lens we are using to test these hypotheses.
Workflow Automation Usability
We believe users will adopt our AI-led automation solution if we create a seamless integration with their existing tools because reducing friction in adoption is key to increasing user satisfaction.
Test: Conduct a smoke test by building a simple integration demo that connects with commonly used tools (e.g., Excel, Salesforce). Invite users to try the integration and observe their ease of use and feedback.
Why smoke testing?: It’s a quick way to validate whether this integration drives user interest without investing heavily in development. If the integration is cumbersome, we know early on that we’ll need to rethink how we structure it.
Value Proposition for Mid-Market
We believe mid-market businesses will pay for our workflow automation solution if we offer a flexible, tiered pricing model because these businesses need customisation but operate with limited budgets.
Test: Launch a landing page or mock-up pricing tiers and use a click-through test to measure interest. Track how many users express interest or sign up for more information based on the pricing structure.
Why click-through testing?: This lightweight approach helps us gauge whether our pricing model resonates with a key target segment without building the full pricing infrastructure. If there’s low interest, we can iterate on the model before committing resources.
AI-Powered Workflows for Large Enterprises
We believe large enterprises will value AI-powered automation if we demonstrate efficiency gains in specific workflows because enterprises are focused on reducing operational costs.
Test: Run a pilot project with a select group of large enterprise customers to automate one specific workflow (e.g., invoice processing). Measure efficiency improvements (time saved, error reduction) and gather feedback on whether the results are compelling enough to encourage broader adoption.
Why a pilot?: Pilots give real-world insights into how enterprises perceive the value of AI. This method lets us test whether the solution fits with their operations before scaling the offering.
Customer Support Scaling
We believe we can scale customer support efficiently if we implement AI-driven support bots because automating common queries will free up human support for higher-value interactions.
Test: Deploy an AI chatbot in a controlled environment, offering it to a subset of customers to handle simple queries. Measure response accuracy, user satisfaction, and support ticket resolution time.
Why use a controlled deployment?: This allows us to validate the bot’s effectiveness in handling queries without overwhelming our human support team. If it works, we scale; if it doesn’t, we refine it before a broader roll-out.
Now that we’ve tested our assumptions, it’s time to synthesise the results and prioritise the solutions we want to bring forward into our MVP. The MVP isn’t about building everything at once — it’s about building just enough to learn and iterate rapidly.
Here’s how we prioritise:
High-Value, Low Complexity: Focus first on solutions that deliver high value to customers while being relatively simple to implement. These are the easiest wins and will help create immediate traction.
Example: A basic AI workflow automation with key integrations (e.g., Excel or Salesforce).
High-Value, High Complexity: These solutions should follow, but only after validating key hypotheses with simpler versions. High complexity doesn’t mean we ignore them, but we need more confidence before investing in the build.
Example: A fully customisable AI-powered workflow solution for large enterprises.
Low-Value, Low Complexity: While tempting to add these “quick wins,” it’s important to ask whether they really move the needle. If not, they may be distractions.
Example: Cosmetic features that don’t directly contribute to core functionality.
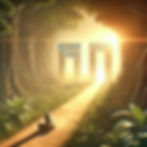
As we emerge from the Hypotheses to Test Jungle, we can start to feel the excitement build. Each test, each validation, brings us closer to something real. The MVP is no longer a distant concept — it’s tangible, informed by real customer feedback, tested solutions, and prioritised features.
The MVP may be minimal by design, but it’s maximally valuable for learning and refining. It’s the beginning of the real journey — the one that moves from planning to execution, from hypothesis to reality, and from luxurious problem space to the world of actual customers.
As we build towards this MVP, the focus shifts from exploration to execution. This is where the fun really begins.